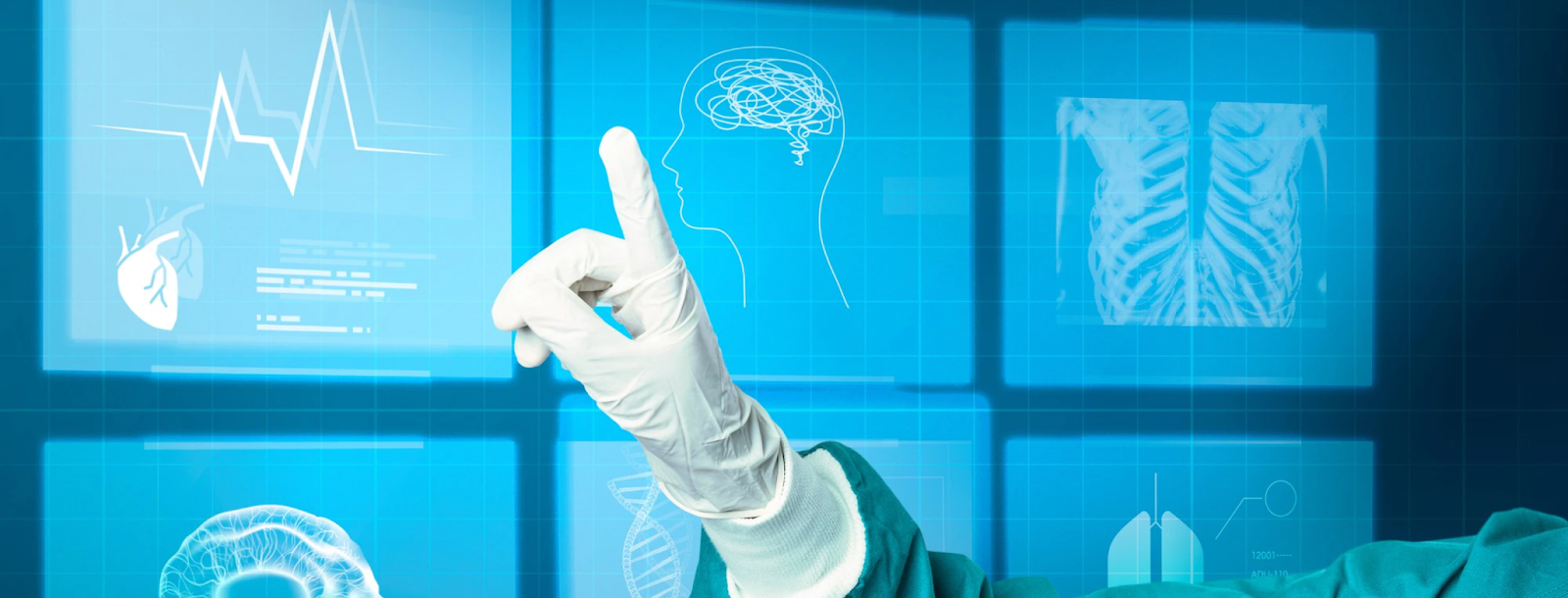
Smart Healthcare: Uncover the Opportunities Through Deep Learning
Deep learning is breaking through the limitations and introducing the era of the smart healthcare industry. Its magic has bridged the gaps and improved the overall healthcare quality of services. Explore the applications of Deep Learning today.
Last Updated On : 09 May, 2023
5 min read
Table of Contents
The Healthcare industry has entered a new era in which biomedical data and technology are treated as a gold mine, digging deeper into it to yield fortunes. For instance, precision medicine guarantees that the practitioner can administer the appropriate therapy to the right patient at the right time by considering numerous elements of patient data. This data involves variability in molecular features, environment, electronic health records (EHRs), and lifestyle.
The vast pool of biomedical data has opened various opportunities, such as introducing deep learning in healthcare. Deep learning, a branch of machine learning (ML), has had a spectacular renaissance over the last six years, partly owing to computational power gains and the availability of enormous new datasets. The discipline has seen significant breakthroughs in machines' capacity to interpret and modify data, including pictures, language, and speech.
Thus, healthcare and medicine stand to profit greatly from deep learning. The driving force is the sheer volume of data created, i.e., 150 exabytes or 1018 bytes in the United States alone, expanding 48% yearly, according to the Stanford Medicine 2017 Health Trends Report. In addition, the rapid proliferation of medical gadgets and digital record systems is revolutionizing the sector from the opposite end.
The Chronicles of Deep Learning
It is a buzzword in the tech world, often slipping off the tongues of tech geeks and industrial lovers. However, only a few have a comprehensive understanding of its concepts. To feed your brain without entangling in the spiderweb of big and complex words, read below:
Deep learning is a subset of machine learning that is essentially a three- or more-layered neural network. These neural networks seek to imitate the activity of the human brain, albeit with limited success, allowing it to "learn" from enormous volumes of data.
A single-layer neural network produces approximate predictions. In contrast, more hidden layers can assist in optimizing and tuning for accuracy.
Diverse artificial intelligence (AI) apps and services rely on deep learning to boost automation by executing analytical and physical activities without human interaction. Deep learning technology is at the heart of both commonplace products and services.
To demonstrate, digital assistants, voice-enabled TV remotes, and credit card fraud detection have popularized our ecosystem. In addition, upcoming innovations, such as self-driving cars, are going to fuel its fame in various industries.
Applications of Deep Learning in Healthcare
With these bold figures and facts, dive into the opportunities for Deep Learning in the Healthcare System.
Precision oozes from the Data Collection Process
Healthcare professionals have the critical and sensitive task of collecting data about patients’ medical history with high precision. It is a challenging task because he is not a data specialist who has extensive knowledge of data entry and processing for managerial and record-keeping purposes. Thus, there is a high risk of human errors, which can lead to the wrong diagnosis.
When such bad days occur, IT applications of Deep Learning in Healthcare emerge as a shiny armor. Healthcare practitioners can use machine learning to capture a patient's history properly.
To do so, this star-stunned technology can also assist in choosing the most pertinent questions to ask a patient depending on various parameters. Afterward, the practitioners can utilize smart healthcare software to predict the most likely scenarios.
Moreover, Deep learning Healthcare software aids patients with restricted mobility in improving their everyday life. It offers the following patient-centric features:
- Smart reminders and scheduling assistance
- Forecasting and preventing probable accidents by recognizing frequent barriers,
- Calculating the optimal pathways
- Receiving support as soon as feasible
Boosting the Quality of Healthcare Services
The major purpose of ML-assisted platforms is to improve the experience of the greatest number of people in healthcare services. However, the ultimate goal of conventional organizations is to maximize profit.
On the other hand, powerful deep learning technologies for hospital operations management must distinguish themselves from traditional systems by integrating empathy with a profit-generating goal.
Furthermore, the pharmaceutical industry increasingly turns to deep learning techniques to overcome the infuriatingly difficult challenge of successful medicine development.
Many new businesses use ML systems to assess multi-channel data utilizing the most advanced reinforcement learning and natural language processing techniques.
The primary aims are to identify patterns and construct high-dimensional representations that can be kept in the cloud and used in the drug-discovery process.
Recommended: The Future of Artificial Intelligence in Healthcare
Enhance the Quality of the Treatment Process
Inadequate insights and erroneous conclusions result when the concerned person mixes the fragmented, duplicated, or missing with hundreds of handwritten document scans. As a result, before ML algorithms can optimally use the data, it must first be properly structured and cleaned.
Once organized, the brilliance of deep learning algorithms enhances the quality of patient-and-doctor engagement. They give physicians daily recommendations on which patients are more likely to be readmitted.
You can also entertain the luxury of having wide-spectrum approaches and treatments that can lessen the risk of sickness in your patients, thanks to algorithms.
The wait is over! Explore our extensive guides to Healthcare Industry
Timely Detection of Disease is a Bounty
Diabetes is a poison that leaves people gasping for their lives and causes a slew of other dreadful disorders. Its venomous strings take hold of your kidney, heart, and nervous system. Then, we have the liver.
It is a key player in metabolism and is susceptible to chronic hepatitis, liver cancer, and cirrhosis. Diagnosing liver disorders is tricky, especially using vast volumes of biomedical data.
Diabetes and liver areas generate a large amount of data based on laboratory evaluations, patient reports, therapy, follow-ups, medicine, etc. It is tough to combine all of the data manually. Because of poor data management, the quality of data organization has suffered.
Increased data volume necessitates the development of an appropriate method for extracting and processing data efficiently and effectively. With the inception of deep learning in medicine, physicians can ensure the timely detection of diabetes and liver disorders. Benefits include reduced burden for medical practitioners and a lower likelihood of human error.
These deep learning tools simulate why and under what conditions diseases are more likely to occur. They notify the practitioners to intervene when the symptoms show up.
Also, they find correct therapeutic alternatives for a person based on their medical history, lifestyle choices, genetic data, and continually changing pathological tests.
Furthermore, researchers now have access to massive amounts of data made public by patients themselves. This will be the source of future advancements in ML in Medicine and make near-perfect predictions. It enables healthcare providers to make helpful ideas on time, estimate disease risk, and assign the appropriate treatment resources.
Also, read about the Benefits of Automation in Healthcare Industry
Smarten up the Online Appointment Scheduling
With an aging population, increasing need for chronic care, transportation barriers, and constrained public and private healthcare budgets, it's no surprise that healthcare providers are always under pressure to improve efficiency.
AI-based appointment scheduling solutions offer a clever way out of the spiderweb. This software can bridge the gap between efficiency and timely access to healthcare.
Deep learning algorithms and techniques examine clinician calendars and assign an appointment date and time. They handle billing and payment, record filing, patient recommendations, reminders, redirect emergency calls, and more.
The Future of Deep Learning In Healthcare
Deep learning in healthcare is on the rise. However, this field is still understaffed. Although companies are not actively hiring professionals with deep learning skills, these resources can become beneficial for organizations in the future that aim to be competitive.
Having said that, partner with InvoZone to earn early access to deep learning specialists. We have experts with sheer knowledge and years of experience under their belt to build and transform your healthcare services and improve customer satisfaction.
Frequently Asked Questions
How can deep learning be used in healthcare?
A DL algorithm may 'learn' to identify correlations and connections in data by evaluating how data is filtered through an ANN's layers and how the layers interact with one another. These features make DL algorithms cutting-edge technologies with the potential to transform healthcare.
What is the difference between Machine Learning and deep learning
Machine Learning refers to computers learning from data and utilizing algorithms to execute a task that has not been explicitly programmed. Deep learning employs a complicated set of algorithms based on the human brain. This allows unstructured data such as documents, photos, and text to be processed.
How AI and deep learning are changing the healthcare industry?
AI can bring value by automating or supplementing physicians' and staff's jobs. Many monotonous processes will be totally automated, and we will be able to employ AI and deep learning to help health professionals perform better at their professions and enhance patient outcomes.
What are the applications of deep learning in the healthcare industry
Deep learning in healthcare is used for disease diagnoses, appointment scheduling, clinical trials, patient data analysis, medicine discovery, radiology, forecasting, and prediction, detecting minor diseases, and more.
Where is deep learning mostly used today?
Deep learning is used across various industries such as chatbots, self-driving cars, medical imaging, fraud detection, and more.
Don’t Have Time To Read Now? Download It For Later.
Table of Contents
The Healthcare industry has entered a new era in which biomedical data and technology are treated as a gold mine, digging deeper into it to yield fortunes. For instance, precision medicine guarantees that the practitioner can administer the appropriate therapy to the right patient at the right time by considering numerous elements of patient data. This data involves variability in molecular features, environment, electronic health records (EHRs), and lifestyle.
The vast pool of biomedical data has opened various opportunities, such as introducing deep learning in healthcare. Deep learning, a branch of machine learning (ML), has had a spectacular renaissance over the last six years, partly owing to computational power gains and the availability of enormous new datasets. The discipline has seen significant breakthroughs in machines' capacity to interpret and modify data, including pictures, language, and speech.
Thus, healthcare and medicine stand to profit greatly from deep learning. The driving force is the sheer volume of data created, i.e., 150 exabytes or 1018 bytes in the United States alone, expanding 48% yearly, according to the Stanford Medicine 2017 Health Trends Report. In addition, the rapid proliferation of medical gadgets and digital record systems is revolutionizing the sector from the opposite end.
The Chronicles of Deep Learning
It is a buzzword in the tech world, often slipping off the tongues of tech geeks and industrial lovers. However, only a few have a comprehensive understanding of its concepts. To feed your brain without entangling in the spiderweb of big and complex words, read below:
Deep learning is a subset of machine learning that is essentially a three- or more-layered neural network. These neural networks seek to imitate the activity of the human brain, albeit with limited success, allowing it to "learn" from enormous volumes of data.
A single-layer neural network produces approximate predictions. In contrast, more hidden layers can assist in optimizing and tuning for accuracy.
Diverse artificial intelligence (AI) apps and services rely on deep learning to boost automation by executing analytical and physical activities without human interaction. Deep learning technology is at the heart of both commonplace products and services.
To demonstrate, digital assistants, voice-enabled TV remotes, and credit card fraud detection have popularized our ecosystem. In addition, upcoming innovations, such as self-driving cars, are going to fuel its fame in various industries.
Applications of Deep Learning in Healthcare
With these bold figures and facts, dive into the opportunities for Deep Learning in the Healthcare System.
Precision oozes from the Data Collection Process
Healthcare professionals have the critical and sensitive task of collecting data about patients’ medical history with high precision. It is a challenging task because he is not a data specialist who has extensive knowledge of data entry and processing for managerial and record-keeping purposes. Thus, there is a high risk of human errors, which can lead to the wrong diagnosis.
When such bad days occur, IT applications of Deep Learning in Healthcare emerge as a shiny armor. Healthcare practitioners can use machine learning to capture a patient's history properly.
To do so, this star-stunned technology can also assist in choosing the most pertinent questions to ask a patient depending on various parameters. Afterward, the practitioners can utilize smart healthcare software to predict the most likely scenarios.
Moreover, Deep learning Healthcare software aids patients with restricted mobility in improving their everyday life. It offers the following patient-centric features:
- Smart reminders and scheduling assistance
- Forecasting and preventing probable accidents by recognizing frequent barriers,
- Calculating the optimal pathways
- Receiving support as soon as feasible
Boosting the Quality of Healthcare Services
The major purpose of ML-assisted platforms is to improve the experience of the greatest number of people in healthcare services. However, the ultimate goal of conventional organizations is to maximize profit.
On the other hand, powerful deep learning technologies for hospital operations management must distinguish themselves from traditional systems by integrating empathy with a profit-generating goal.
Furthermore, the pharmaceutical industry increasingly turns to deep learning techniques to overcome the infuriatingly difficult challenge of successful medicine development.
Many new businesses use ML systems to assess multi-channel data utilizing the most advanced reinforcement learning and natural language processing techniques.
The primary aims are to identify patterns and construct high-dimensional representations that can be kept in the cloud and used in the drug-discovery process.
Recommended: The Future of Artificial Intelligence in Healthcare
Enhance the Quality of the Treatment Process
Inadequate insights and erroneous conclusions result when the concerned person mixes the fragmented, duplicated, or missing with hundreds of handwritten document scans. As a result, before ML algorithms can optimally use the data, it must first be properly structured and cleaned.
Once organized, the brilliance of deep learning algorithms enhances the quality of patient-and-doctor engagement. They give physicians daily recommendations on which patients are more likely to be readmitted.
You can also entertain the luxury of having wide-spectrum approaches and treatments that can lessen the risk of sickness in your patients, thanks to algorithms.
The wait is over! Explore our extensive guides to Healthcare Industry
Timely Detection of Disease is a Bounty
Diabetes is a poison that leaves people gasping for their lives and causes a slew of other dreadful disorders. Its venomous strings take hold of your kidney, heart, and nervous system. Then, we have the liver.
It is a key player in metabolism and is susceptible to chronic hepatitis, liver cancer, and cirrhosis. Diagnosing liver disorders is tricky, especially using vast volumes of biomedical data.
Diabetes and liver areas generate a large amount of data based on laboratory evaluations, patient reports, therapy, follow-ups, medicine, etc. It is tough to combine all of the data manually. Because of poor data management, the quality of data organization has suffered.
Increased data volume necessitates the development of an appropriate method for extracting and processing data efficiently and effectively. With the inception of deep learning in medicine, physicians can ensure the timely detection of diabetes and liver disorders. Benefits include reduced burden for medical practitioners and a lower likelihood of human error.
These deep learning tools simulate why and under what conditions diseases are more likely to occur. They notify the practitioners to intervene when the symptoms show up.
Also, they find correct therapeutic alternatives for a person based on their medical history, lifestyle choices, genetic data, and continually changing pathological tests.
Furthermore, researchers now have access to massive amounts of data made public by patients themselves. This will be the source of future advancements in ML in Medicine and make near-perfect predictions. It enables healthcare providers to make helpful ideas on time, estimate disease risk, and assign the appropriate treatment resources.
Also, read about the Benefits of Automation in Healthcare Industry
Smarten up the Online Appointment Scheduling
With an aging population, increasing need for chronic care, transportation barriers, and constrained public and private healthcare budgets, it's no surprise that healthcare providers are always under pressure to improve efficiency.
AI-based appointment scheduling solutions offer a clever way out of the spiderweb. This software can bridge the gap between efficiency and timely access to healthcare.
Deep learning algorithms and techniques examine clinician calendars and assign an appointment date and time. They handle billing and payment, record filing, patient recommendations, reminders, redirect emergency calls, and more.
The Future of Deep Learning In Healthcare
Deep learning in healthcare is on the rise. However, this field is still understaffed. Although companies are not actively hiring professionals with deep learning skills, these resources can become beneficial for organizations in the future that aim to be competitive.
Having said that, partner with InvoZone to earn early access to deep learning specialists. We have experts with sheer knowledge and years of experience under their belt to build and transform your healthcare services and improve customer satisfaction.
Frequently Asked Questions
How can deep learning be used in healthcare?
A DL algorithm may 'learn' to identify correlations and connections in data by evaluating how data is filtered through an ANN's layers and how the layers interact with one another. These features make DL algorithms cutting-edge technologies with the potential to transform healthcare.
What is the difference between Machine Learning and deep learning
Machine Learning refers to computers learning from data and utilizing algorithms to execute a task that has not been explicitly programmed. Deep learning employs a complicated set of algorithms based on the human brain. This allows unstructured data such as documents, photos, and text to be processed.
How AI and deep learning are changing the healthcare industry?
AI can bring value by automating or supplementing physicians' and staff's jobs. Many monotonous processes will be totally automated, and we will be able to employ AI and deep learning to help health professionals perform better at their professions and enhance patient outcomes.
What are the applications of deep learning in the healthcare industry
Deep learning in healthcare is used for disease diagnoses, appointment scheduling, clinical trials, patient data analysis, medicine discovery, radiology, forecasting, and prediction, detecting minor diseases, and more.
Where is deep learning mostly used today?
Deep learning is used across various industries such as chatbots, self-driving cars, medical imaging, fraud detection, and more.
Share to:
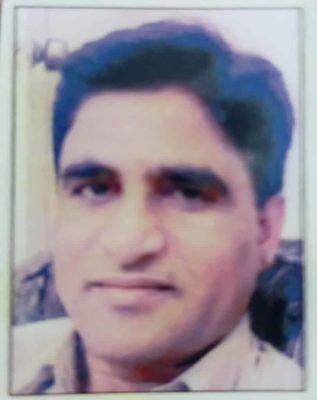
Written By:
Abdul KarimAbdul Karim brings to the table years of experience as a marketing campaign specialist. Re... Know more
Get Help From Experts At InvoZone In This Domain