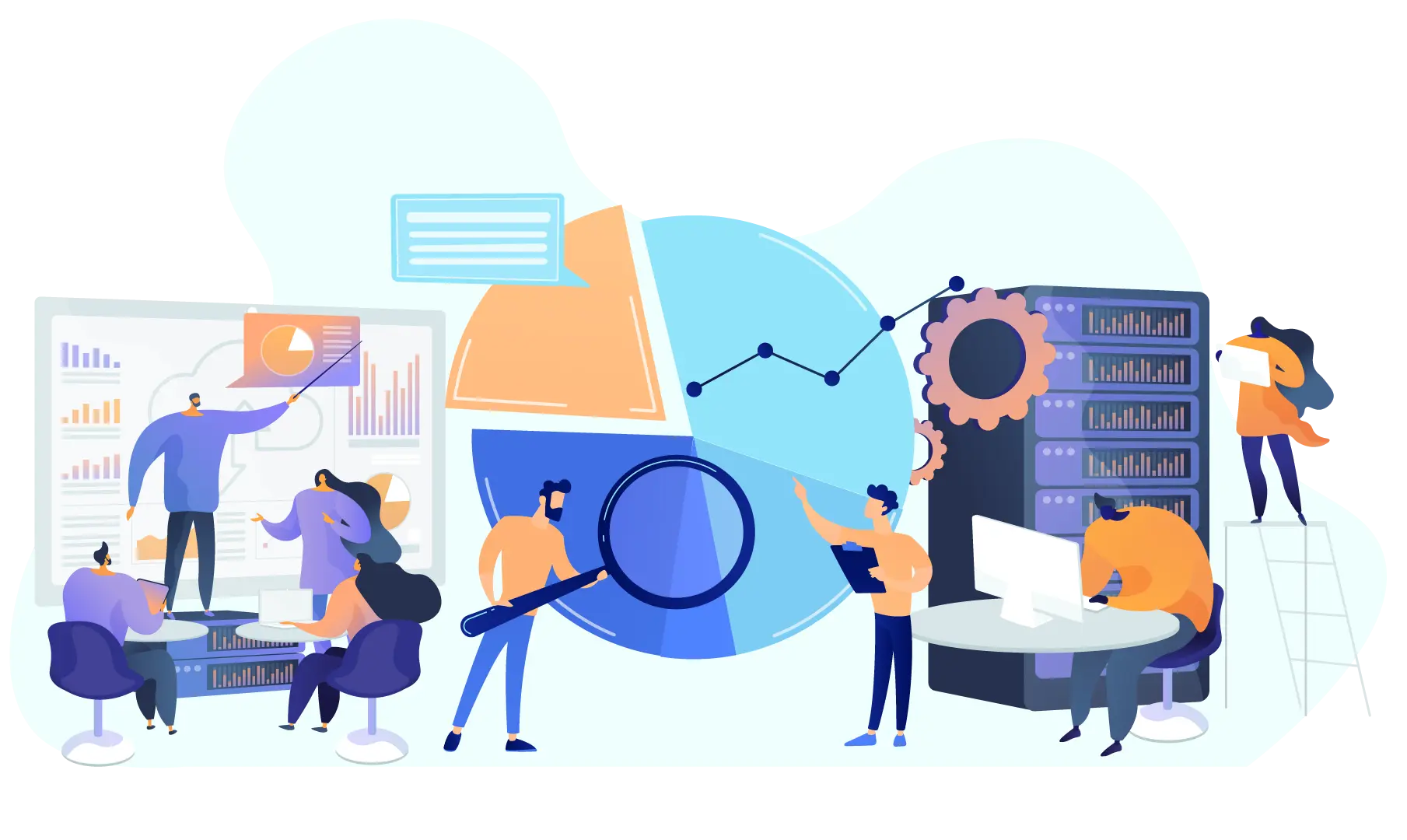
Top 6 Big Data Analytics Challenges Faced By Enterprises And How To Solve Them
In this article, we'll discuss how companies can solve the top 6 big data analytics challenges.
Let's dive in!
Last Updated On : 16 June, 2023
3 min read
Table of Contents
Collecting data and deciphering information from it has evolved with human civilization. From prehistoric data storage that used tally sticks to today’s sophisticated data analytics technologies of Hadoop and MapReduce, we have come a long way in analyzing and storing data.
You’ll be surprised to know that the amount of data produced by large enterprises is increasing by 40% to 60% per year. In fact, 2.5 quintillion bytes of data are produced every day.
This doesn’t stop here. International Data Corporation (IDC) previously estimated that the amount of data generated will double every two years. Needless to say that the amount of data generated every second is staggering and we need techniques to analyze and understand this massive data. As it is a great source to derive useful information.
Advanced data analytics can be used to transform big data into smart data for obtaining critical information regarding large data sets. Smart data provides actionable insights and helps in improving decision-making capabilities for organizations in multiple industries.
For instance, in healthcare, analytics performed on big data sets gathered from Electronic health records and clinical decision systems may enable healthcare practitioners to deliver effective and affordable healthcare solutions for patients. This can be done by examining trends in the overall patient history instead of relying on evidence provided by localized data or current data.
However, it is difficult to perform analysis on big data using traditional analytics. Because this big data loses effectiveness due to the five V's characteristics of big data: High Volume, Low Veracity, High Velocity, High Variety, and High Value.
To resolve this analytical problem, numerous artificial intelligence techniques including machine learning (ML), deep learning (DL), natural language processing (NLP), data mining, and computational intelligence were designed. These techniques are fast, accurate, and precise.
In this article, we’ll discuss, what big data analytics challenges businesses face today and how to solve them.
What Big Data Analytics Challenges Enterprises Face Today?
Companies often get stuck at the initial stage of their big data analytics projects. This is mostly because they are not aware of the challenges that come with big data. Let us explore these challenges one by one and discuss how to solve them.
Also Read: BIG DATA: A NEW FOCAL POINT OF HEALTHCARE AND LIFE SCIENCES
1. Lack of Proper Understanding of Big Data Analysis
To make data useful, it is important to analyze the huge volume of data produced each second. The demand for big data scientists and big data analysts has been created in the market with the exponential rise of data. And business often stays reluctant to acquire these skills. This inadequate knowledge often leads to no or wrong analysis which is of no use.
To overcome this challenge, businesses need to understand what big data analytics is. And one of the best ways to do this is to arrange basic big data analytics training programs for every level of the organization.
2. Data Growth Issues
One of the most pressing challenges in big data analytics is storing huge sets of data properly. The amount of data stored in data centers and databases is increasing rapidly. As these data sets grow exponentially with time, it becomes extremely difficult to handle and analyze.
Most of this data is in unstructured formats and comes from different sources such as documents, videos, audio, and text files. This means it can not be fit in a single database and analyzed properly.
To store the growing data, leading enterprises are opting for modern techniques, such as compression, tiering, and deduplication. The process of compressing is used for reducing the number of bits in the data, thus reducing its overall size.
Deduplication is used to remove duplicate and unwanted data from a data set. Data tiering allows businesses to store data in different storage tiers. It guarantees that the data is kept in the best possible storage space. For this purpose, companies use tools like Hadoop and NoSQL.
3. Confusion while Selecting Big Data Tools
When choosing the appropriate technology for storing and analyzing large data, businesses often experience confusion. For example, choosing between HBase or Cassandra for data storage or selecting the best between Hadoop MapReduce and Spark for data analytics. Choosing the wrong technology for any task in the data analytics pipeline may result in a loss of money, time, and effort.
The best way to go about this is to get professional help. You can either hire professional big data engineers who have expertise in working with these tools. Another way is to go for complete Big Data consulting. The big data consultants will recommend you the perfect tools based on your company’s scenario. With this advice, you can work out a strategy and select the best tools for you.
4. Lack of Data Professionals
To run these modern data analytics techniques, companies need skilled data professionals. These professionals often include data scientists, data analysts, and data engineers who are experienced in working with these tools and making sense of huge data sets.
Unfortunately, many companies face the problem of an acute shortage of data professionals. This is mostly because of the rapidly increasing amount of data.
To overcome this challenge, companies with high budgets are investing money in the recruitment of skilled professionals. However, for companies that can’t afford to hire in-house professionals and provide training to them, employees other ways such as developing data analytics solutions powered by artificial intelligence techniques or hiring remote dedicated teams already having ample knowledge and expertise.
5. Data Security
Securing huge data sets is one of the ominous challenges of big data that has been under discussion for years. Companies often put data security to later phases because they are so busy understanding, storing, and analyzing their data sets. But this move could lead to unprotected data repositories which are breeding grounds for malicious hackers.
Several data breach incidents have been recorded in the past and the most disturbing part is that even big companies are not safe from these attacks. Organizations can lose millions for a stolen record or data breach.
Companies need to cater to data security problems in parallel with the storage and analysis of data by following practices:
- Data encryption
- Identity and access control
- Implementation of endpoint security
- Data segregation
- Real-time data monitoring
6. Integrating Data From Multiple Sources
For organizations, data may come from a variety of sources, such as social media pages, ERP applications, customer logs, financial reports, emails, and reports created by employees.
Combining all this data to get insights is a challenging task. Data integration is crucial for analysis and decision making so it has to be perfect.
Companies can integrate data by using the right tools. Some of these integration tools are:
- Talend Data Integration
- Centerprise data integrator
- IBM Infosphere
- Xplenty
To put big data to the best use, companies have to change their strategies. This means hiring professional staff, changing management, and reviewing existing policies and technologies being used.
Reference:
Uncertainty in big data analytics: survey, opportunities, and challenges
Don’t Have Time To Read Now? Download It For Later.
Table of Contents
Collecting data and deciphering information from it has evolved with human civilization. From prehistoric data storage that used tally sticks to today’s sophisticated data analytics technologies of Hadoop and MapReduce, we have come a long way in analyzing and storing data.
You’ll be surprised to know that the amount of data produced by large enterprises is increasing by 40% to 60% per year. In fact, 2.5 quintillion bytes of data are produced every day.
This doesn’t stop here. International Data Corporation (IDC) previously estimated that the amount of data generated will double every two years. Needless to say that the amount of data generated every second is staggering and we need techniques to analyze and understand this massive data. As it is a great source to derive useful information.
Advanced data analytics can be used to transform big data into smart data for obtaining critical information regarding large data sets. Smart data provides actionable insights and helps in improving decision-making capabilities for organizations in multiple industries.
For instance, in healthcare, analytics performed on big data sets gathered from Electronic health records and clinical decision systems may enable healthcare practitioners to deliver effective and affordable healthcare solutions for patients. This can be done by examining trends in the overall patient history instead of relying on evidence provided by localized data or current data.
However, it is difficult to perform analysis on big data using traditional analytics. Because this big data loses effectiveness due to the five V's characteristics of big data: High Volume, Low Veracity, High Velocity, High Variety, and High Value.
To resolve this analytical problem, numerous artificial intelligence techniques including machine learning (ML), deep learning (DL), natural language processing (NLP), data mining, and computational intelligence were designed. These techniques are fast, accurate, and precise.
In this article, we’ll discuss, what big data analytics challenges businesses face today and how to solve them.
What Big Data Analytics Challenges Enterprises Face Today?
Companies often get stuck at the initial stage of their big data analytics projects. This is mostly because they are not aware of the challenges that come with big data. Let us explore these challenges one by one and discuss how to solve them.
Also Read: BIG DATA: A NEW FOCAL POINT OF HEALTHCARE AND LIFE SCIENCES
1. Lack of Proper Understanding of Big Data Analysis
To make data useful, it is important to analyze the huge volume of data produced each second. The demand for big data scientists and big data analysts has been created in the market with the exponential rise of data. And business often stays reluctant to acquire these skills. This inadequate knowledge often leads to no or wrong analysis which is of no use.
To overcome this challenge, businesses need to understand what big data analytics is. And one of the best ways to do this is to arrange basic big data analytics training programs for every level of the organization.
2. Data Growth Issues
One of the most pressing challenges in big data analytics is storing huge sets of data properly. The amount of data stored in data centers and databases is increasing rapidly. As these data sets grow exponentially with time, it becomes extremely difficult to handle and analyze.
Most of this data is in unstructured formats and comes from different sources such as documents, videos, audio, and text files. This means it can not be fit in a single database and analyzed properly.
To store the growing data, leading enterprises are opting for modern techniques, such as compression, tiering, and deduplication. The process of compressing is used for reducing the number of bits in the data, thus reducing its overall size.
Deduplication is used to remove duplicate and unwanted data from a data set. Data tiering allows businesses to store data in different storage tiers. It guarantees that the data is kept in the best possible storage space. For this purpose, companies use tools like Hadoop and NoSQL.
3. Confusion while Selecting Big Data Tools
When choosing the appropriate technology for storing and analyzing large data, businesses often experience confusion. For example, choosing between HBase or Cassandra for data storage or selecting the best between Hadoop MapReduce and Spark for data analytics. Choosing the wrong technology for any task in the data analytics pipeline may result in a loss of money, time, and effort.
The best way to go about this is to get professional help. You can either hire professional big data engineers who have expertise in working with these tools. Another way is to go for complete Big Data consulting. The big data consultants will recommend you the perfect tools based on your company’s scenario. With this advice, you can work out a strategy and select the best tools for you.
4. Lack of Data Professionals
To run these modern data analytics techniques, companies need skilled data professionals. These professionals often include data scientists, data analysts, and data engineers who are experienced in working with these tools and making sense of huge data sets.
Unfortunately, many companies face the problem of an acute shortage of data professionals. This is mostly because of the rapidly increasing amount of data.
To overcome this challenge, companies with high budgets are investing money in the recruitment of skilled professionals. However, for companies that can’t afford to hire in-house professionals and provide training to them, employees other ways such as developing data analytics solutions powered by artificial intelligence techniques or hiring remote dedicated teams already having ample knowledge and expertise.
5. Data Security
Securing huge data sets is one of the ominous challenges of big data that has been under discussion for years. Companies often put data security to later phases because they are so busy understanding, storing, and analyzing their data sets. But this move could lead to unprotected data repositories which are breeding grounds for malicious hackers.
Several data breach incidents have been recorded in the past and the most disturbing part is that even big companies are not safe from these attacks. Organizations can lose millions for a stolen record or data breach.
Companies need to cater to data security problems in parallel with the storage and analysis of data by following practices:
- Data encryption
- Identity and access control
- Implementation of endpoint security
- Data segregation
- Real-time data monitoring
6. Integrating Data From Multiple Sources
For organizations, data may come from a variety of sources, such as social media pages, ERP applications, customer logs, financial reports, emails, and reports created by employees.
Combining all this data to get insights is a challenging task. Data integration is crucial for analysis and decision making so it has to be perfect.
Companies can integrate data by using the right tools. Some of these integration tools are:
- Talend Data Integration
- Centerprise data integrator
- IBM Infosphere
- Xplenty
To put big data to the best use, companies have to change their strategies. This means hiring professional staff, changing management, and reviewing existing policies and technologies being used.
Reference:
Uncertainty in big data analytics: survey, opportunities, and challenges
Share to:
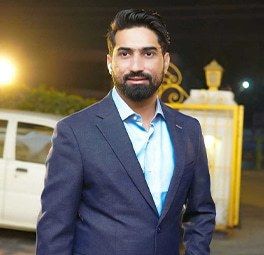
Written By:
Furqan AzizFurqan Aziz is CEO & Founder of InvoZone. He is a tech enthusiast by heart with 10+ years ... Know more
Get Help From Experts At InvoZone In This Domain